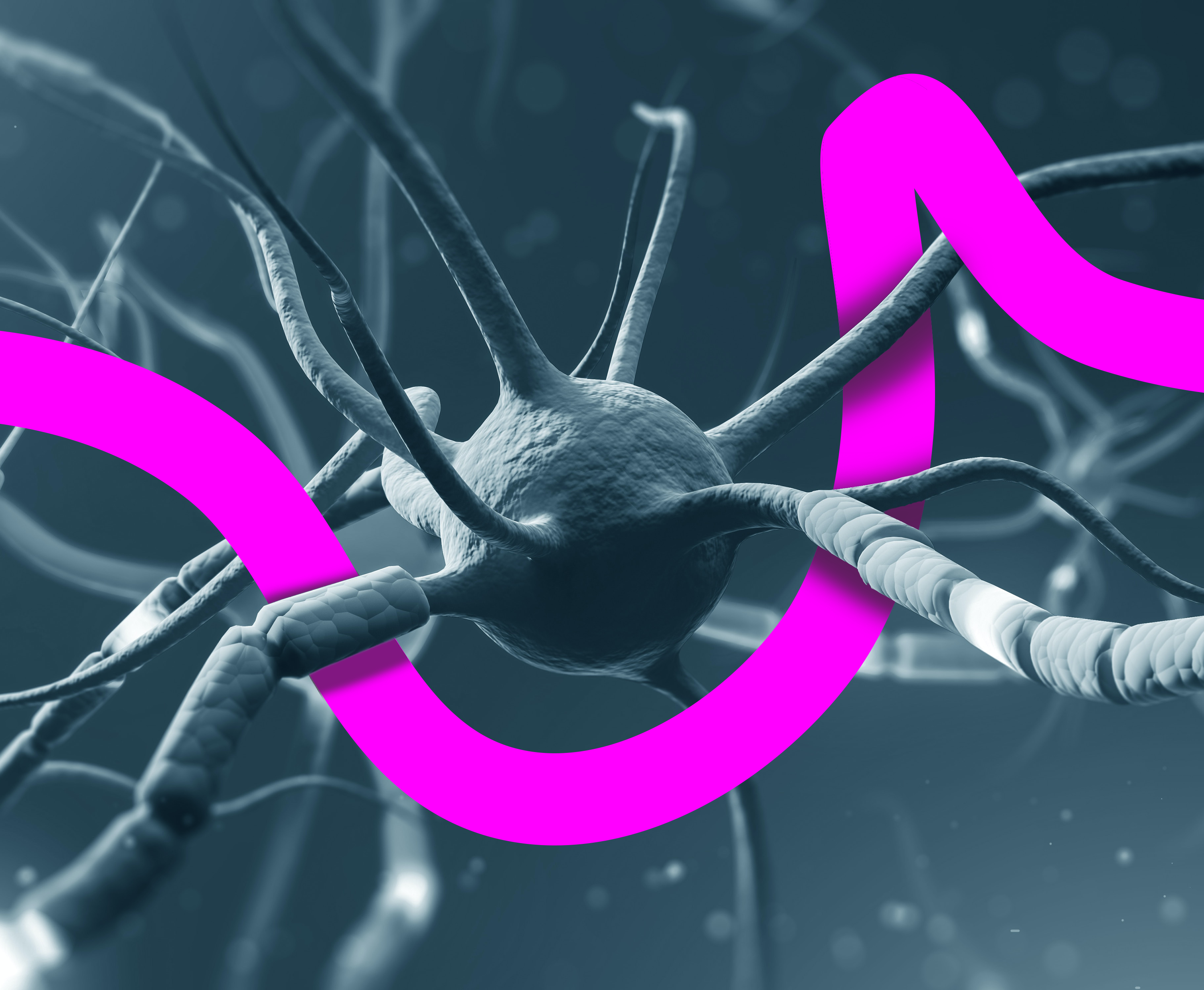
5 ways to use generative AI for social listening
Generative AI has well and truly arrived with promises of changing the way we work for the better - we’ve already seen a lot of social listening technology providers integrate some form of generative AI into their tools. And whilst many claim that generative AI can help us to analyse data and uncover insights more quickly, the reality is that the technology is not yet advanced enough to do this alone (and might not be for some time). It still requires us humans to intervene to generate true value from social data.
Having said that, there are some ways in which generative AI can certainly make our lives easier. Here are five examples shared by social listening professionals during this year’s Demo Day.
1. Summarising large amounts of data
We all know that the amount of social data available to analyse is huge and ever-growing. That means the amount of information we humans need to ingest and understand is also growing. For searches around niche themes, this might be possible to do manually. However, for big, mainstream topics, it’s almost impossible to read every single post to understand the key themes and conversations that are happening around it.
This is where generative AI can be really effective, as it’s been designed to analyse vast amounts of data and identify patterns quickly. As a result, if you’re just looking to understand the basic ideas being shared in conversations around a particular theme, it can provide quick summaries for you. This allows you to then focus your time on the fun bit: extracting the insights from the data.
Martin Miliev, Vice President of Social Intelligence at Publicis Groupe, uses generative AI for this type of work and was able to catch-up on conversations around COVID. “I had several searches during COVID that I’d forgotten about…it provided a great summarisation of all the stuff that happened over the last eight months that I haven’t kept an eye on the topic.”
2. Understanding historical context
A key use case for social listening is to really understand our customers interests and pain points in order to develop more useful products. For this, we often have to analyse historical conversations to learn how their relationship with the product or the challenge it solves has changed over time. This can involve analysing a LOT of data to find trends. Again, this is something that generative AI is great at - identifying patterns from large data sets.
This is one way Chris Chen, Executive Director of Global Social Intelligence at Warner Bros. Discovery has used it. When his company works on developing new storylines for any of their major franchises, it’s crucial that the right characters are included and that the relationships between all the characters hold true. As Chris explains about the DC franchise, “Our characters have been around for a very long time…so there are a lot of stories, a lot of team-ups and a lot of rivalries. The problem is that all of this is now part of the characters’ histories and we’re trying to understand, if we’re going to make a film, or a TV series or an animation, what are those characters that need to be involved. Batman is not much without his Bat Family…which one of his Bat Family is important to him, and why are they important? Those are things that ChatGPT can help us with. Then we can really utilise social listening to really dive into the details about why they care.”
3. Researching keywords
One of the (arguably) less interesting but time-consuming tasks of a social listening professional is finding the right keywords to include in a query to ensure you get the most relevant results. And sometimes you need it to return the broadest set of results possible. In both cases, it requires you to think differently and put yourself in the minds of a target audience to understand how they might think about or refer to a specific topic.
Generative AI, such as ChatGPT, can add real value here, as it has been trained on public data sets and therefore has an understanding of the different ways people might refer to a certain object, food or topic, including the unusual ones that you might not be aware of. This means it will likely identify more relevant keywords than you will ever be able to on your own.
Melissa Davies, Senior Manager of Global Insights Excellence at Mondelez International explains that she uses generative AI for this type of research regularly. “I once asked Chat GPT for a list of words that people might use to describe the texture of chocolate. I got back a list of around 25 words…and probably the first ten were ones that were already on my list, but there were some additional words and terms that I hadn’t thought of. It was helpful to get that bit of extra input, and it was certainly faster to do it that way.”
Natália Leão, Global Social Intelligence Development Lead and Nestlé and one of the winners of our social listening query writing competition, also used ChatGPT to research keywords for the competition. She needed to find insights on over-65’s snacking trends. “ChatGPT suggested the idea of expressing seniors' ages in written form (e.g., sixty-four instead of just 64) or using the term "treat" in reference to snacks, which I hadn't considered previously.”
4. Writing boolean queries
After identifying keywords,the next crucial step is writing the social listening queries themselves. And again, this is something that can be quite time consuming, often requiring several iterations to perfect.
Several people at Demo Day have been using generative AI to help at this stage too. For example an audience member, Ben Ellis, Social Intelligence Researcher and Consultant, told us “I mainly use [GitHub] Copilot in Visual Studio to help with query writing. I use AI to help me with lengthy, multi-language queries (e.g., finding social handles and brand hashtags for several brands in just a couple seconds). Once I'm happy with the queries, I can then add them to the social listening tool(s) I'm using.”
However, for the boolean queries to return accurate results, they need well-written prompts. And that requires some level of research beforehand. Whether that is keyword research, or understanding the question to be answered and the context around it. So, whilst it can make the actual writing of a query quicker, it might not speed up the process of designing them. As Martin highlights, ”We’re probably going to spend as much time running prompts as we used to run boolean searches.”
5. Working with foreign languages
Finally, in the globalised times we live in, it’s not uncommon that the audience you want to gather insights about will speak a different language to your own. But to get a complete picture of your audience, it’s also important to understand their conversations. In that sense, generative AI can really help you not only to summarise non-English posts, as Martin explains - “I use it for Bulgarian and had several searches running during COVID” - but also to understand the individual posts and videos that are being shared. It can also help you to find keywords and create queries in languages that you don’t speak.
This is something that Natália took advantage of when creating her query for the SI Lab competition. She was able to discover new keywords using ChatGPT. “It was particularly valuable because I didn’t have a team to brainstorm with, and being a non-native English speaker, it has served as a helpful ‘project buddy’.”
Generative AI is great…but has limitations
As we’ve seen, there are many ways that generative AI can support our work as social listening practitioners, and as technology in this area develops it’s likely to take even more burdensome tasks off our hands. But, as with every new technology that emerges, it needs to be used with caution.
At this stage, to ensure the accuracy of the results that generative AI is giving, human intervention is still required. For tasks that we know it can help us with, it’s still important to manually review the outputs and to check the original sources it references to ensure the information and data that it provides is accurate before you start looking for insights. Because insights generated from bad data aren't insights at all. And, they could have negative consequences.
And there are still many tasks that generative AI can’t do as well as humans. In particular insights generation. It may well be able to do this type of work in future but, until then, it’s important that social listening teams develop processes that enable them to identify when generative AI can be used and add value, and when human input is necessary.
This interview was recorded via LinkedIn Live, if you prefer to view on LinkedIn, click the button below.
View InterviewSee related content

.png)
