.jpg)
Developing processes for using generative AI in social listening: what it can and can't do
As generative AI currently dominates so much of the discourse around work life, it’s become impossible to ignore. In fact, at this year’s Demo Day, it was a theme that ran through much of the programme, from technology providers showcasing how they’re incorporating it into social listening tools, to practitioners discussing how they’re using it as part of their daily work. And it’s unsurprising, given the many advantages it’s promised to bring in terms of cost and time savings.
So, should we be embracing generative AI technologies wholeheartedly as an industry? Or are there dangers we need to be aware of before we throw away our traditional methods of social data analysis?
As we discussed with our panel of experts at Demo Day, for it to add true value to our work we need to understand what generative AI can reliably do, and what its limitations are. And from there, we should develop processes to ensure we can use this new technology responsibly and effectively.
What is generative AI in the context of social listening?
The use of AI in social listening is nothing new. One of its advantages is the ability to analyse vast amounts of data, identify patterns and make decisions or predictions based on that analysis.
Until recently, AI has been used for specific tasks such as image recognition and language processing: the core functionalities of most traditional social listening tools. Now, however, generative AI is being incorporated into many social listening technologies. This type of AI is classified as a type of general AI which aims to mimic human intelligence across a wide range of tasks. This means - in theory - it can quickly understand and analyse large data sets to the extent that it can provide accurate summaries, offer suggestions around inputs, and share insights from the analysis.
Already we’ve seen several social listening vendors promoting their tool’s generative AI functionalities, some of which showcased them at Demo Day this year.
For example, YouScan was one of the first to introduce generative AI through their ChatGPT-powered conversation assistant, Copilot. This enables natural language querying of social data (i.e., you can ask questions in plain English) to get data summarisation, explanations about specific trends, SWOT analyses and insights into particular audiences.
ViralMoment, a specialist in social video, has incorporated computer vision to watch and comprehend entire videos and learn to see and understand trends. They’ve also incorporated a natural language chatbot into the platform to answer user questions.
Runic is another platform that uses generative AI, building their entire product on it. By training its models on specific use cases, it can generate more accurate analysis and insights than you would using just keywords. Some of the areas they focus on are accurately analysing brand names, and finding ‘needles in haystacks’., i.e. extracting relevant insights from massive data sets. For example, they have worked with a new social network, Spoutible, to monitor conversations on Meta’s Threads and X (formerly Twitter) - a task that would be incredibly difficult if using just keywords.
How is generative AI currently being used for social listening?
And beyond the tools, social listening practitioners are starting to incorporate generative AI within their daily work. During the panel on this topic at Demo Day, we discussed the different ways it can help social data analysts.
One of the main ways is to summarise large amounts of data. This is particularly relevant for searches on topics that return many results. For example, Martin Miliev, Vice President of Social Intelligence at Publicis Groupe, used it to catch-up on conversations around COVID. “I had several searches during COVID that I’d forgotten about…it provided a great summarisation of all the stuff that happened over the last eight months that I haven’t kept an eye on the topic.” Chris Chen, Executive Director of Global Social Intelligence at Warner Bros. Discovery also uses it for this type of work. For him, this function is particularly useful given the industry he works in. People are passionate about film and are clear about what they love and hate, so it’s important for him to know the many thousands of different conversations that are happening around this topic. “Whilst we can utilise tools to help us filter down to key things that we’re trying to understand, when I’m analysing millions of posts at a time, that summarisation does help quite a bit.”
Another area that it’s being used is in keyword research. As Melissa Davies, Senior Manager of Global Insights Excellence at Mondelez International explained, “I’ve used ChatGPT to help me think through the process of building keywords that I might need or ways to slice the data…we’re always playing the game of making sure the keyword set includes not just what I think or how I would describe something, but also how other consumers would talk about it.”
Other practitioners are also using generative AI to help with writing boolean queries, particularly with lengthy, multi-language queries that might involve finding different social handles and brand hashtags.
What are its limitations currently?
With all of these uses, however, everyone agreed that human intervention is needed to ensure the quality of the results that are returned. And that highlights some of the limitations of generative AI.
The main one being that it struggles to reliably analyse data and extract meaningful insights. According to Martin, “it works fine to summarise the data, but it can’t go beyond the data. It works with what it’s given and most of the time that’s the text. And as analysts, we have many more senses because we can look at the people’s profiles, history of posts and get a much better understanding of what’s happening.” Chris agreed, highlighting that “we as analysts need to provide the additional context…to expand upon the summary so that we can actually tell the story so the marketers can do something with that information.”
Another area of concern is around the accuracy of the results generative AI returns, even when doing tasks such as summarising data. As Melissa explains, “whenever I think about some of the results of something that comes from AI analysis, there are moments when I think ‘yes, spot on’ and there are moments when I think, ‘hmm..not quite’ and I feel that way about generative AI too…It’s helpful, but it still needs that human interpretation to make sure that we’re getting away from the hallucinations and it really is what it says it is.”
And even the social listening tools themselves highlight that there are challenges with this, and are including guardrails to reduce the risks. For example, YouScan’s Copilot includes links to the original sources of its results so that you can verify that they aren’t made up. And Runic, as they introduce natural language querying, are hard coding prompts to avoid hallucinations.
The unreliability of results, though, can result in a lack of trust in generative AI for social data analysis. As Melissa highlighted, “We can’t send out unscreened research findings that miss the boat a little bit and then have our business make decisions based on something that’s not quite right or contextualised.”
How to develop processes to incorporate generative AI responsibly
The reason for these limitations are because of three key challenges with generative AI generally, according to Ali Maaxa, media anthropologist and Founder of Maaxa Labs. Firstly, it’s not great at context, secondly it lacks critical thinking and thirdly it isn’t able to do embodied thinking, i.e. understand how the body works and changes over time and how to bring this sensory world into its analysis.
As a result, for generative AI to be incorporated successfully into the practice of social listening, humans need to add the elements that this technology can’t. As Ali explains, we all need to think, “what is the job we’re using ChatGPT for, where do humans intervene, where are those handoffs and where can we refine those according to our specific needs and contexts?”
This is largely because the broader infrastructure to support generative AI in a business setting isn’t available yet. Until that happens though, it’s important to develop frameworks of how to use it within your organisation. That means sitting down as a team to map out, end-to-end, the work that needs to be done, where generative AI can be used to reduce workloads, and where human intervention is essential - for example in writing prompts, reviewing the results for accuracy, and using critical thinking to make judgements on results that are nuanced (for example sarcasm). According to Ali, “by taking that tool and thinking of it as an end-to-end process rather than a drop-in tool, you have all the agency in the world to enter it in.”
This does mean that, for the time being at least, working with generative AI may not be a quicker route to generating insights. Even for something like writing boolean queries, it still requires a thoroughly researched, well-written prompt. As Martin highlights, ”We’re probably going to spend as much time running prompts as we used to run boolean searches.”
Is generative AI a useful tool for social listening professionals?
Despite the rise of generative AI within social listening technology that promises to make our lives easier by generating accurate insights from social data more quickly, the current reality is slightly different. Whilst many practitioners agree that there is a strong use case for it supporting the inputs of social data analysis - i.e. query writing and topic research - there is still a long way to go until they fully trust the outputs that these tools give.
And this is largely due to the fact that the technology itself is not ready to do some of the more advanced and nuanced jobs that data analysis requires. As Ali points out, ”when we’re using them as part of our operations [as opposed to consumers testing it out], especially part of operations that are complex, multi-person jobs to be done, there’s a lot more complexity there. And the language and the lexicon just isn’t in place yet. It’s really up to us to put it in place.”
But the technology will continue to develop and improve, and in the meantime there are processes that social listening practitioners can put in place that enable them to use generative AI in a way that helps, rather than hinders their work. According to Ali, “we have so little control over how the tools are engineered…but we do have the agency within our own organisations to say that if we’re going to use the tools we build an infrastructure around it.”
This interview was recorded via LinkedIn Live, if you prefer to view on LinkedIn, click the button below.
View InterviewSee related content
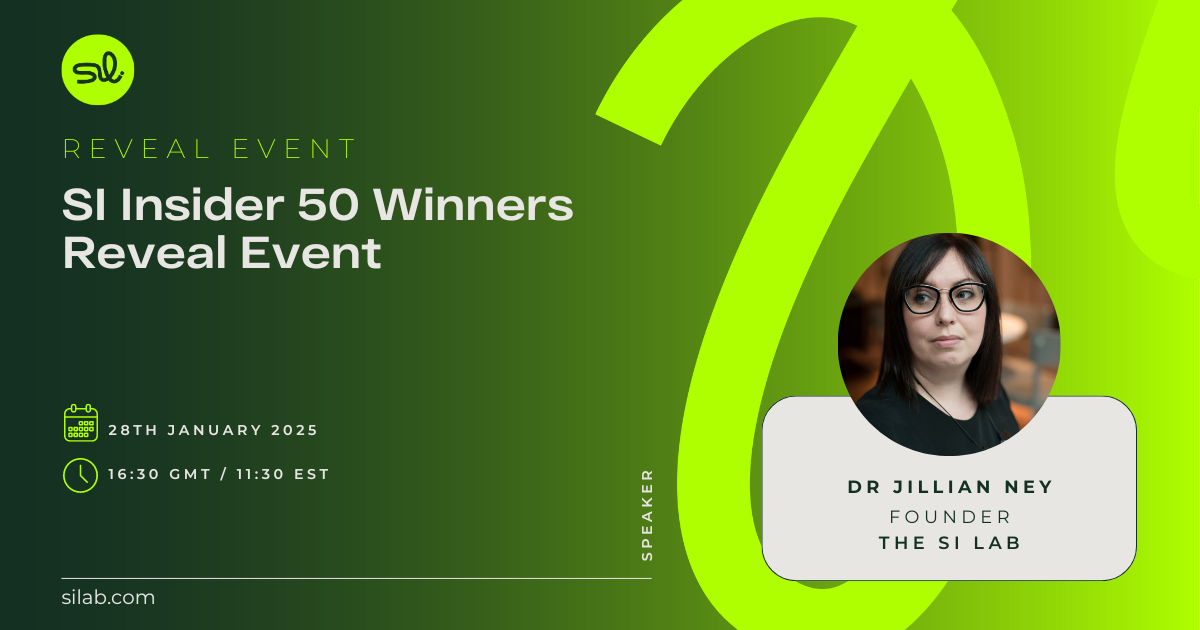

.png)