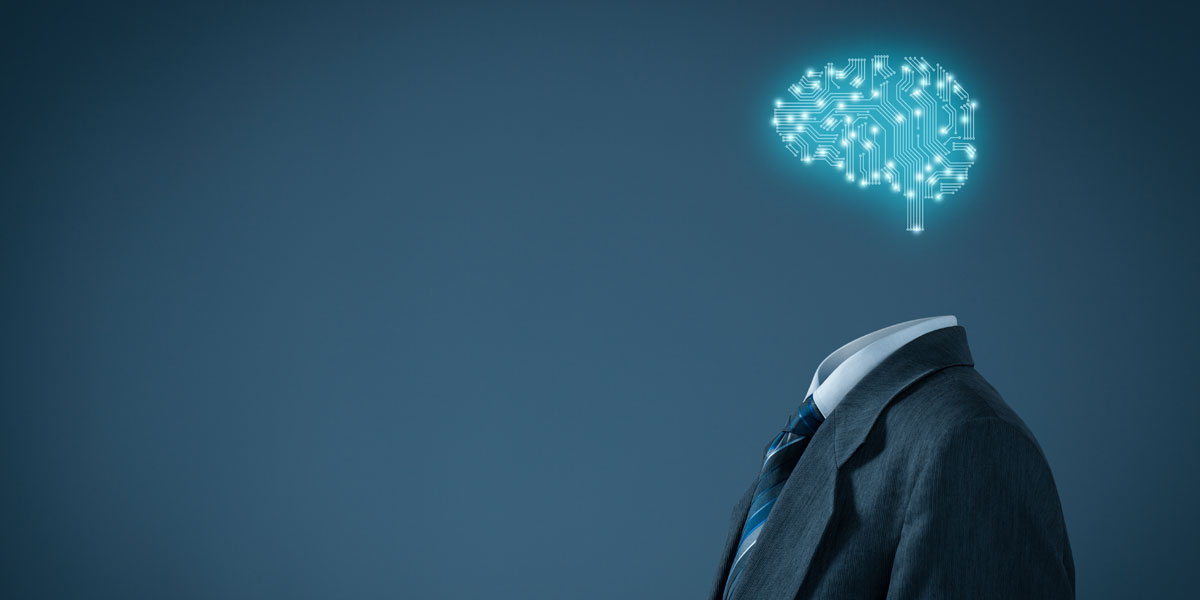
The journey from social listening to enterprise decision intelligence
For over a decade and a half now, social listening data has held great promise for researchers and communication professionals seeking more rapid, unprompted insights. But the promise has been stymied by the reality of general poor data quality and methodology limitations.
Analyzing unstructured data properly is a challenge
Indeed, for years, unstructured “conversation data” – whether through social or other sources like customer feedback and reviews – has represented a massive and growing resource that has consistently been under leveraged by brands. According to Forrester Research, organizations are only analyzing approximately 21% of their unstructured data. And for some good reasons. Conversation data is generated through human language – and human language is complex. Slang, sarcasm and implicit meanings dominate the data. Add to that, as much as 70-80% of data generated and shared in social platforms is not driven by actual “humans,” but instead by bots, news media, job listings, advertisements and other sources.
Effectively taming and transforming this massive data source into “research grade” data that can be used with confidence for critical enterprise insight needs has required significant technical and methodological innovation, much of which has only emerged fairly recently. The practice of computational linguistics – or natural language processing – is more than 60 years old but it is only recently that algorithms are able to process and analyze data “as humans do” even when certain keywords are not present and the language is nuanced and complex. Getting to this point requires several important processes that transcend the largely pervasive “one size fits all” algorithmically constrained general “sentiment analysis” that has, (some good reasons) given rise to skepticism of automated approaches. Add to that, not all organizations see the world in the same way; they require most customized data categorization and measurement approaches that align to their definitions, industry and structures.
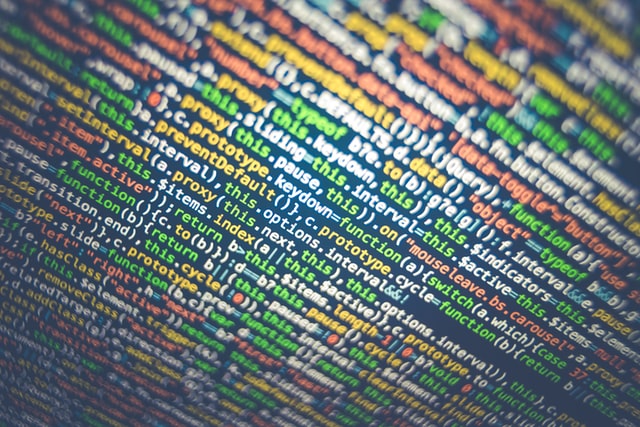
Unlocking insight requires an applied AI approach
Unlocking the full insight value of this data requires going beyond most standard AI approaches and embrace an applied AI approach that is focused on practical problem solving and aligns models with critically important “frameworks.” For example, if you set loose three individuals, without guidance, to annotate records that they consider expressions of “trust,” you are going to get three drastically different results. Trust may mean different things to different people, and without demonstrate definitive definitions and frameworks, each individual will filter their annotations based on their own personal experiences and biases. Even for a bit more basic “sentiment analysis,” we have found that individuals often only agree with each other about 60-80% of the time.
Applied AI approaches start with a clear understanding of the task at hand, develop essential definitions and coding guidelines, and apply a wisdom of the crowds approach so that multiple labellers are looking at, and reviewing, the same content with appropriate intercoder adjudication for when there is wide divergence. But it is equally important that these “crowds” are not general public per se, but instead apply subject matter expertise to the process. And throughout this process, it’s critical that humans remain in the loop via a semi supervised approach.
With this type of approach we are now able to begin classifying data with great sophistication and nuance that goes far beyond “sentiment” into critical areas like “trust”, “advocacy” “detraction” and more. And the data classifications can be adjusted to specific brands and use cases. High quality pre-built models now available can also accelerate the process and reduce the costs and burdens to data science teams. Basic general emotions can give way to specific “tonalities” that bring even more insight such as “frustration”, “aggrieved" etc). Models can also be used to filter data to actual human voices (eliminating that 70-80% of noise in many categories).
A one-size-fits-all approach will fall short
An important lesson here is that all models need to be aligned to specific domains and industries. Language differs by industry (the word "small" is good for smart phones but not so great for hotel rooms, for example). One-size-fits-all approaches such as those that come as default in most basic “listening” and customer feedback platforms are not designed for that purpose.
Furthermore, with the application of auto ML performance testing, consumers of these technologies can now remove the black box and understand exactly how well each model performs in the wild before using – through automated diagnostics and scoring such, as F1.
An applied AI approach provides the foundation for predictive insights
This is where it becomes exciting for the analytics community. The rigorous and accurate data from these models can then be combined, configured and leveled up together against comprehensive, multi-dimensional measurement frameworks, such as “customer experience”, where functional and emotional classification can get to truly accurate, meaningful and actionable analysis. Brand relevance frameworks can demonstrate how well your product and brand attributes align with the unprompted (and often changing) expressed needs of key consumers, and even allow you to discover attributes, drivers and topics that you didn’t know to look for through organic topic discovery. These classifications extend even further to critical areas such as brand purpose, corporate citizenship (reflecting ESG frameworks), brand reputation and much more, with rich and robust variables.
This applied AI approach provides the critical foundation for the next evolution of this space: transforming insights from reactive and descriptive to predictive. One knock on social data has been that it might not be representative and many of the metrics, while interesting, can be considered vanity metrics by skeptics. It has been a fair criticism since the data and insights had not necessarily been fully proven out, until fairly recently.
One of the benefits of social data is its ability to time travel and get real actionability. The better we understand the past, the greater we can predict the future. Through this applied NLP approach, the data is not just cleansed and classified with multiple variables; advanced analytics modeling has demonstrated that it, indeed, can be anchored to critical business outcomes. At Converseon, we have teams that have deep expertise in combining this conversation data with first party sales data, for example, and, depending on category, have been able to predict sales up to six months ahead. The reputation measurement approaches using this data have also been shown in peer reviewed studies to explain shareholder value and provide a breakdown of the dimensions within the reputation framework that most align to that outcome. And driver analysis can pinpoint even further those essential areas that explain why things are happening, and what you can do about it. Since it's public data, this scoring can be compared to competitors and industry leaders and laggards for gap analysis. There is now a comprehensive body of evidence that demonstrates, definitively, the powerful predictive nature of this data which has opened the doors for greater adoption of this data among sophisticated analytics groups at innovative organizations.
Looking to the future
This predictive component provides the inputs for the next opportunity: creating powerful decision intelligence “simulators”, that provide clear guidance on what specific actions an organization can take to drive revenue or reduce costs, (or both). For example through modeling we could demonstrate that a reduction in complaints by X, can correlate to an increase in repeat purchase by Y. This provides actionable, quantitative insight that can make a real impact on an organization's bottom line. It also provides an important route to explain the specific, tangible and quantitative value (or attribution) that public relations and reputation management provide.
The evolution from “social listening” to social intelligence to enterprise decision intelligence has been long in development but is now accelerating quickly. The combination of auto NLP and AI, together with proven measurement methodologies and frameworks, and the blending of this data with other first party and related data, provides an essential contribution to an "always on" system of insight that is rigorous, predictive and prescriptive in a polarized era where real time sometimes isn’t fast enough.
This interview was recorded via LinkedIn Live, if you prefer to view on LinkedIn, click the button below.
View InterviewSee related content
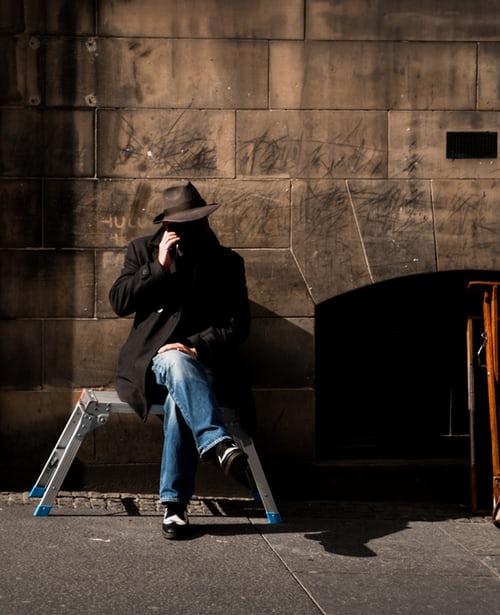

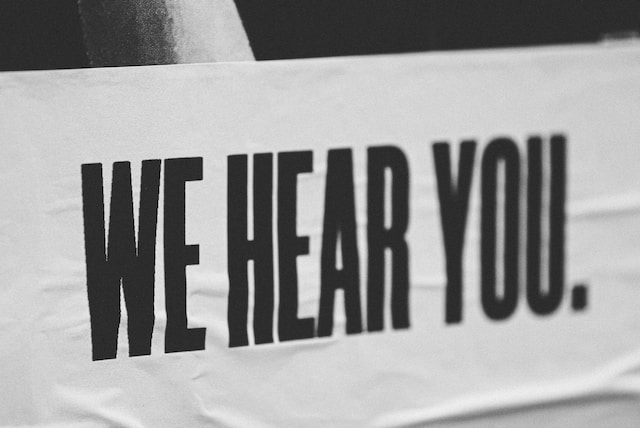