
Using AI to measure ‘intangibles’ in social media
Rule-based analytics has always been a part of the traditional social listening process. But there’s only so much you can learn from it, especially when it comes to ‘intangibles.’
But what are ‘intangibles’ in social media? And how do we measure them?
During the SI Tech Demo Day 2021, Ben Sigerson, VP of Solutions at Converseon gave us some insights into the world of measuring social media ‘intangibles.’ Converseon specializes in AI-powered social media and consumer intelligence, developing solutions to transform unstructured social media and customer data into “research-grade data.”
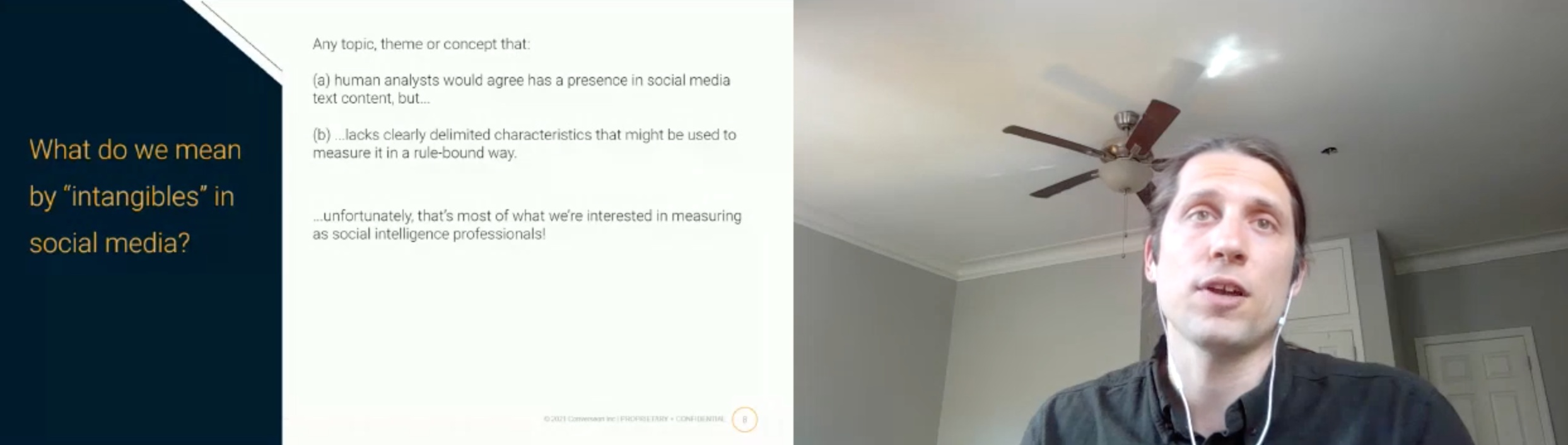
What are ‘intangibles’ in social media?
According to Ben, ‘intangibles’ refer to the topics, themes, and concepts that people don’t explicitly talk about or express but rather through implicit statements. This makes them challenging to measure using traditional, rule-bound methods, such as Boolean queries, and searches can result in false positives and false negatives.
For example, if you want to measure brand trust, it’s hard to get clear insights by using a boolean search query based on variations of the word ‘trust’. People rarely use this word explicitly when referring to the trustworthiness of a brand. So, with this search query, you’re likely to miss a lot of valuable insights.
How do we measure intangibles?
The best way to measure intangibles is using an AI and machine learning (ML) model. This can pick up on the nuances of natural language and capture relevant content with much better accuracy than keyword-based queries.
Ben explains that, even in a single linear machine learning model, you may have over half a million distinct words, phrases and grammatical concepts. All of these go into the model’s “final learned understanding of the intangible concept that it’s trying to identify.” This is what helps to achieve accuracy.
But of course, to make sure that your model isn’t just performing anecdotally well but also across thousands of social posts, you need a quick and reliable validation process.
Auto ML platforms like Conversus from Converseon makes this possible, allowing you to “ address validation as a part of the larger model training and deployment workflows.”
Validating the model gives confidence that you’ll get a very high fidelity set of data to report on. So, you can be sure that when you apply the model to 5 million social media posts about 20 different brands in a particular industry, you’re going to get a signal back that’s relevant to the intangible concept you’re trying to identify.
What’s the value of measuring intangibles?
To help us get an even better understanding of why we should measure intangibles, Ben also took us through some case studies. In one, a global food company wanted to use social media conversations to predict future sales growth in the vegan food industry.
Converseon applied AI-powered emotion classification and AI-powered classification for intangible behavioural motivators across 4 years’ worth of social media data. To do this, they built an AI model that captured content where consumers were describing comfort or recognizable ingredients as a motivator for buying or eating vegan food.
These were then trained and tested using the Conversus platform, which helped them establish a 0.82 correlation between social data variables and sales data from the same period. With this, they could predict sales 3-6 months in advance for parts of the vegan food category.
Final thoughts
Measuring intangibles in social media can add an extra layer of accuracy to your social data. Using AI-powered natural language processing and a sophisticated validation process will give you more accuracy in classifying datasets and analyzing sentiment. It also gives you better noise filtering along with more sophisticated topic discovery and measurement.
This interview was recorded via LinkedIn Live, if you prefer to view on LinkedIn, click the button below.
View InterviewSee related content
.png)
.png)
